Guides & best practices
View all articles5 common A/B testing mistakes (and what to do instead)
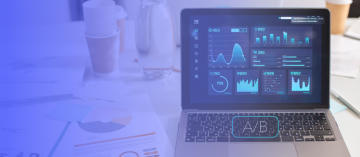
A/B testing is a game-changer for optimizing your sales funnel and boosting conversion rates, but it’s not always smooth sailing. Done right, it can reveal invaluable insights that drive growth. Done wrong, and it can mislead you, wasting time and resources on the wrong changes.
The reality is that many A/B tests don’t yield the results they should because of common, avoidable mistakes. This article will explore these pitfalls and provide practical solutions to avoid them, ensuring your tests provide clear, actionable insights. With the right tools, such as Heyflow’s powerful analytics and testing features, you’ll be able to streamline your A/B testing process for your sales funnel and make smarter decisions for better results.
Mistake 1: Not using actionable insights from past tests
Many teams jump into new tests without reviewing previous test outcomes, leading to redundant or incomplete experiments. Without a clear understanding of what has worked or failed in the past, you risk repeating mistakes or missing opportunities for improvement. Every test you run is a chance to learn more about your audience and refine your strategy, so you shouldn’t waste it on lack of preparation.
To avoid this, it’s essential to review past A/B test results that are relevant to your current context, using their insights to guide your current strategy. By revisiting historical data, you can build on what’s already been tested, making adjustments based on previous results rather than starting from scratch. This will help you understand what has worked for similar funnels, landing pages or audiences, creating a continuous learning loop and allowing you to design experiments with a higher likelihood of success.
Heyflow makes this easier by providing access to historic A/B test results directly in the Analytics dashboard, including key metrics like visitors by device or conversion rate for each variant. This context is crucial for refining your testing strategy and avoiding repeated mistakes.
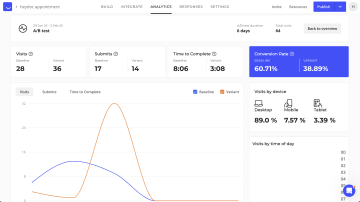
Mistake 2: Testing too many elements at once
When creating a lead funnel, form or landing page, it can be tempting to create two completely different variants that look nothing alike in order to test as many changes as possible, but this often leads to confusion and diluted results. When too many variables are involved, it’s difficult to pinpoint which factor caused the change in performance, making it nearly impossible to extract clear insights from the test.
Instead of overwhelming your test with too many variables, focus on testing one or a few specific changes at a time. This approach provides clearer insights and makes it easier to identify what drives improvements, so you can gather actionable data that can guide your next steps.
If you’re unsure what things to test and need some ideas, check out our blog post on what elements you can A/B test in your heyflow.
Mistake 3: Failing to maintain clean and high-quality data
Data quality is the foundation of any successful A/B test. Compromised data – whether due to setup errors, or issues during the testing phase – can distort results and lead to flawed decisions. For instance, a sudden drop in traffic caused by technical glitches or ad campaign mishaps can produce skewed data, making it difficult to accurately assess the true impact of your changes.
To avoid this, it’s crucial to clean and validate your data. Heyflow simplifies this process, allowing you to reset your test data and start fresh whenever needed. Simply click the “Clear Analytics” button and your A/B test will continue with a clean slate, ensuring only relevant and accurate data informs your decisions.
Beyond cleaning, it’s also important to deeper analyze your gathered data in terms of quality. Heyflow enriches response data with variant information which can be sent to CRMs and other connected tools, allowing you to analyze which variant not only converts better but also brings in higher-quality leads. For example, while Version A might generate more conversions, Version B could get you more valuable responses. This insight is crucial for making informed decisions beyond just the numbers.
Mistake 4: Neglecting traffic allocation between variants
When running A/B tests, many users stick to the default 50/50 traffic split between variants. While this can be a good starting point, it’s not always the most effective strategy, especially for tests with larger or more radical changes. Allocating traffic equally might not be the best option if you’re testing high-risk variations, need a gradual rollout, or want to mitigate risk in case a variant performs poorly.
Adjusting traffic distribution allows you to control exposure and ensure your test results are reliable and meaningful. For example, if you’re testing a major redesign, you might want to start with a smaller portion of traffic directed to the new variant to reduce the risk.
Heyflow allows you to easily adjust the percentage of traffic each variant receives, giving you more control over how your test is executed. Whether you need to roll out changes gradually or want to test a more radical idea, you can fine-tune your traffic allocation based on your test’s specific needs.
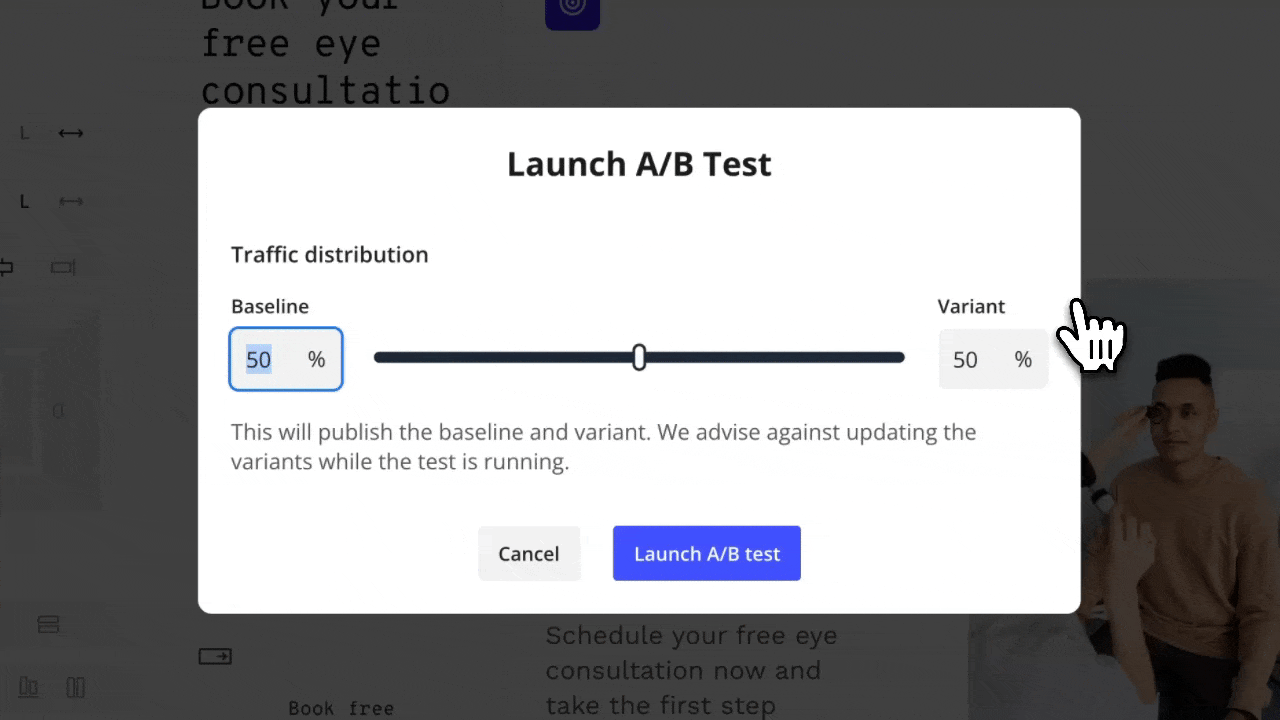
Mistake 5: Ending tests too early, before reaching statistical significance
Jumping to conclusions without fully understanding your test results can lead to poor decision-making. One of the biggest mistakes is ignoring statistical significance and probability when evaluating your results. If you don’t properly assess the likelihood of success, you might mistakenly treat inconclusive results as definitive or make changes based on false positives.
To avoid this, always consider the statistical probability of success when interpreting your test results. This will help you determine whether a variant is truly performing better, or if the results could be due to random chance. By using probability metrics, you can make more confident decisions, reducing the risk of acting on unreliable data.
Heyflow provides A/B test winner probability indicators, helping you assess whether the results are statistically significant. With clear signals on whether the test outcome is definitive or still uncertain, you can make smarter, more confident decisions based on data.
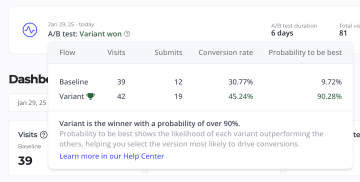
Conclusion
A/B testing is a powerful tool, but it’s important to avoid common pitfalls that can lead to inaccurate results. By learning from past tests, focusing on key elements, maintaining clean data, properly allocating traffic, and interpreting results with statistical significance, you can optimize your testing strategy and boost your conversion rates.
With Heyflow’s upgraded A/B testing features, you’ll be equipped to avoid these mistakes and run tests that provide reliable, actionable insights. Ready to get started?
Start your free trial today and take your A/B testing to the next level.